FFTNet: a Real-Time Speaker-Dependent Neural Vocoder
The 43rd IEEE International Conference on Acoustics, Speech and Signal Processing (ICASSP), April 2018
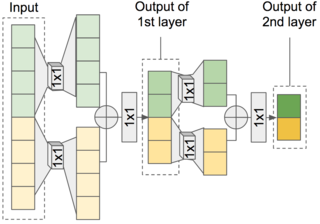
FFTNet architecture: given size-8 inputs, they are first divided into two halves; each passed through a different 1x1 convolution layer and then summed together. The summed size-4 output will pass through ReLU and then another 1x1 convolution and ReLU before repeating the the same operation.
Abstract
We introduce FFTNet, a deep learning approach synthesizing audio waveforms. Our approach builds on the recent WaveNet project, which showed that it was possible to synthesize a natural sounding audio waveform directly from a deep convolutional neural network. FFTNet offers two improvements over WaveNet. First it is substantially faster, allowing for real-time synthesis of audio waveforms. Second, when used as a vocoder, the resulting speech sounds more natural, as measured via a "mean opinion score" test.
Links
- Paper preprint (670kb)
- Listen to audio clips from our experiments.
Citation
Zeyu Jin, Adam Finkelstein, Gautham J. Mysore, and Jingwan Lu.
"FFTNet: a Real-Time Speaker-Dependent Neural Vocoder."
The 43rd IEEE International Conference on Acoustics, Speech and Signal Processing (ICASSP), April 2018.
BibTeX
@inproceedings{Jin:2018:FAR, author = "Zeyu Jin and Adam Finkelstein and Gautham J. Mysore and Jingwan Lu", title = "{FFTNet}: a Real-Time Speaker-Dependent Neural Vocoder", booktitle = "The 43rd IEEE International Conference on Acoustics, Speech and Signal Processing (ICASSP)", year = "2018", month = apr }